Over 8 years ago I completed a Bachelor in Electronics Engineering, with a focus on embedded systems. Since then I have done primarily software engineering in embedded and web projects, sometimes combined in so-called “Internet of Things” (IoT) projects. Often there was a strong data- and signal-processing focus in these systems; from audio processing in microphone-arrays, to image processing for smart website builders. Recognizing the importance of data, I realized around 2 years ago I wanted to add a new skill-set to my engineering capabilities: Data Analysis and Machine Learning (ML).
And today I’m proud to say that I have successfully completed the Master in Data Science program at the Norwegian University of Life Sciences, as one the first batch to have this degree in Norway.
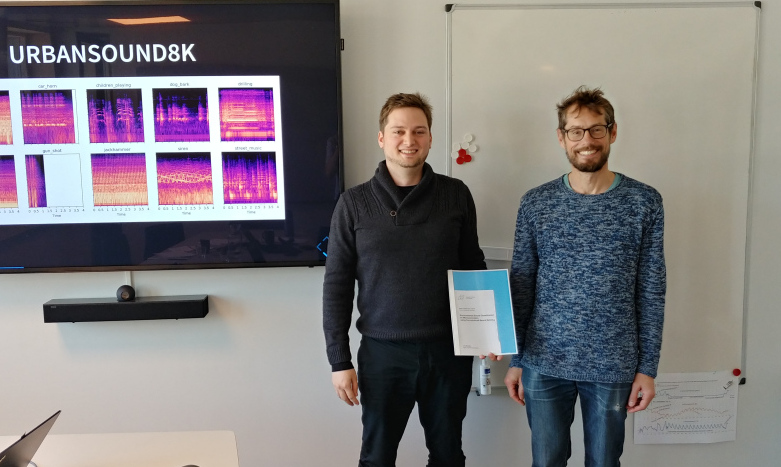
Master of Data Science thesis successfully defended. Left: me, Right: External sensor Lars Erik Solheim
Research
Throughout my degree, I’ve kept the vast majority of my notes in the open-source way – public on Github. Over time I have distilled these into two resources covering the main topics of my work.
Embedded Machine Learning: Machine Learning applied to Embedded System, with a focus on-edge ML in low-cost, low-power sensors.
Machine Hearing: Using Machine Learning on audio, with a focus on general sound (less music and speech).
Thesis
My masters thesis combined these two topics, and applied it to classification of everyday urban sounds for noise monitoring in smart cities. The report and all the code can be found on Github:
Environmental Sound Classification on Microcontrollers using Convolutional Neural Networks
Software
Since Embedded Machine Learning is an emerging niche, the availability of software tools are not as good as for machine learning in general. To help with that I developed emlearn, an open-source ML inference engine tailored for micro-controllers and very small embedded systems. emlearn allows to convert models built with existing Python machine learning frameworks such as scikit-learn and Keras, and execute them on device using portable C code. The focus is on simple and efficient models such as Random Forests, Decision Trees, Naive Bayes, linear models. In this way, emlearn is a compliment to deep learning inference libraries for embedded devices, such as TFLite and X-CUBE-AI.
Consulting
While the master degree was nominally a full time program, I kept doing engineering work for customers in the period. Projects have included:
Windportal. An interactive installation for advertising the Hywind off-shore windmill farms for Equinor. Made with ad-agency Dept and software developer Martin Stensgård.
dlock. A IoT doorlock system for retrofitting existing public infrastructure doors. Developed for municipality of Oslo as part of the Oslonøkkelen project, an app that allows inhabitants to access municipality services such as libraries and recycling stations outside of manned working-hours. Made in collaboration with IoT solutions provider Trygvis IO.
Since the start of this year, I have started to focus on machine learning projects. Especially things that incorporate my particular expertise: Embedded/Edge Machine Learning, Machine Learning for Audio, and Machine Learning on IoT sensor data. The first ML consulting project for Roest coffee is well underway (details to be announced). Going forward, most of my time is dedicated to products at my new startup, Soundsensing. However, there should also be some capacity for new consulting work.